There has been significant advancement in AI and machine learning, particularly with the development of large language models like GPT recently. These models have demonstrated their prowess in harnessing extensive text data to achieve remarkable performance across various natural language understanding tasks. As a result, there is a growing interest in expanding these capabilities to encompass additional modalities such as images, audios, and videos, giving rise to large vision-language models (LVLMs) like GPT-V. LAVA workshop aims to unleash the full potential of research in large vision-language models (LVLMs) by emphasizing the convergence of diverse modalities, including text, images, and video. Furthermore, the workshop provides a platform for delving into the practical applications of LVLMs across a broad spectrum of domains, such as healthcare, education, entertainment, transportation, finance, etc.
- Duc-Tuan Luu, Viet-Tuan Le, Duc Minh Vo: Questioning, Answering, and Captioning for Zero-Shot Detailed Image Caption
- Rento Yamaguchi, Kenji Yanai: Exploring Cross-Attention Maps in Multi-modal Diffusion Transformers for Training-Free Semantic Segmentation
- Viet-Tham Huynh, Trong-Thuan Nguyen, Thao Thi Phuong Dao, Tam V. Nguyen, Minh-Triet Tran: DermAI: A Chatbot Assistant for Skin lesion Diagnosis Using Vision and Large Language Models
- Felix Hsieh, Huy Hong Nguyen, April Pyone Maung Maung, Dmitrii Usynin, Isao Echizen: Mitigating Backdoor Attacks using Activation-Guided Model Editing
- (1st prize) Thanh-Son Nguyen, Viet-Tham Huynh, Van-Loc Nguyen, Minh-Triet Tran: An Approach to Complex Visual Data Interpretation with Vision-Language Models
- (2nd prize) Gia-Nghia Tran, Duc-Tuan Luu, Dang-Van Thin: Exploring Visual Multiple-Choice Question Answering with Pre-trained Vision-Language Models
- (3rd prize) Trong Hieu Nguyen Mau, Binh Truc Nhu Nguyen, Vinh Nhu Hoang, Minh-Triet Tran, Hai-Dang Nguyen: Enhancing Visual Question Answering with Pre-trained Vision-Language Models: An Ensemble Approach at the LAVA Challenge 2024
We welcome people to submit papers about large vision-language models (LVLMs) to LAVA workshop. Accepted papers will be presented in our workshop and will be published in the ACCV workshop proceeding. We accept short papers (non-archived) which are up to 7 pages in ACCV format, excluding references; and long papers (archived) which are up to 14 pages in ACCV format, excluding references. Submission policies adhere to the ACCV submission policies.
The topics in this workshop will include but are not limited to:
- Data preprocessing and prompt engineering in LVLMs
- Training/Compressing LVLMs
- Self-supervised and/or unsupervised, few-/zero-shot learning in LVLMs
- Generative AI
- Trust-worthy/Explainable LVLMs learning
- Security and privacy in LVLMs
- LVLMs evaluation and benchmarking
- LVLMs for downstream tasks
- LVLMs in virtual reality, mixed reality
- Applications of LVLMs
- LVLMs and other modalities
- LVLMs for low resources
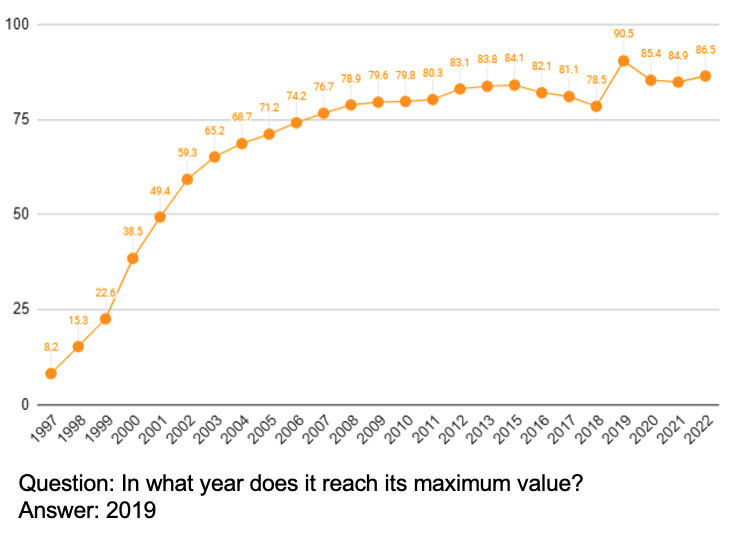
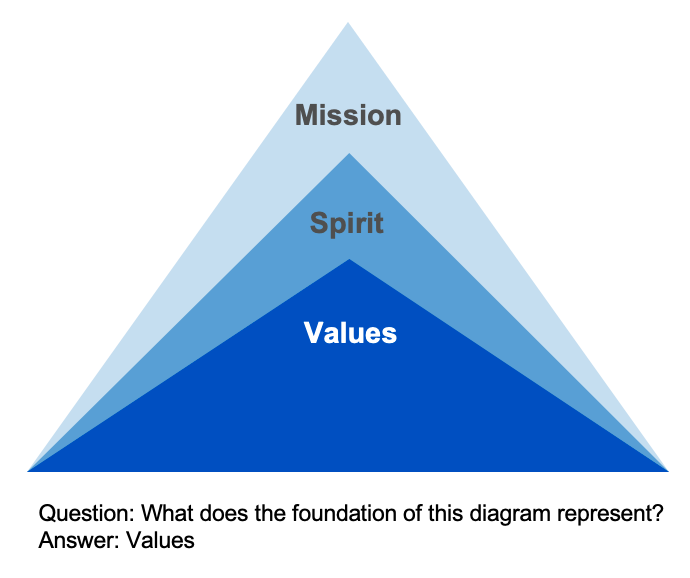
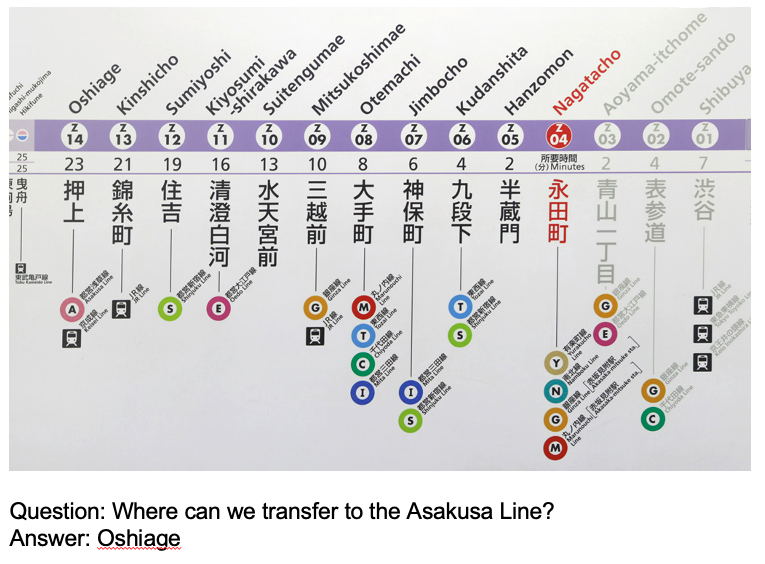
Datasets:
- Public dataset: We will release our dataset collected from the internet. It contains about 3,000 samples.
- Private dataset: The TASUKI team (SoftBank) provides this dataset. It contains about 1,100 samples.
Metric:
We will evaluate using MMMU.
Final score = 0.3 * Public dataset + 0.7 * Private dataset
Results
Team Name | Public score | Privare score | Final score |
---|---|---|---|
WAS | 0.85 | 0.85 | 0.85 |
MMLAB-UIT | 0.83 | 0.84 | 0.84 |
V1olet | 0.82 | 0.82 | 0.82 |
Prizes and Travel Grants:Travel grants are available for winning teams (one per team). Prizes will be announced later.
Computational Resources:Participants from the University of Tokyo may use SoftBank Beyond AI SANDBOX GPUs.
- Challenge track opened: 2024/8/15
- Test set released: 2024/8/30
- Challenge track closed: 2024/9/30
- Regular paper submission deadline: 2024/9/30
- Challenge track paper submission deadline: 2024/10/15
- Acceptance notification: 2024/10/30 2024/10/18
- Camera-ready deadline: 2024/11/15 2024/10/20
- Workshop date: 2024/12/8 (Afternoon)
- 13:30 - Opening Remark
- 13:40 - Keynote Talk 1 - Dr. Asim Munawar (IBM Research, US): "LLM-Based Reasoning: Opportunities and Pitfalls "
- 14:40 - Poster session + Coffee break
- 15:50 - Keynote Talk 2 - Dr. April Pyone Maung Maung (NII, Japan): "Adversarial Attacks and Defenses on Vision-Language Models"
- 16:40 - Challenge Award - Dr. Md Anwarus Salam Khan (Softbank, Japan)
- 16:55 - Closing Remark
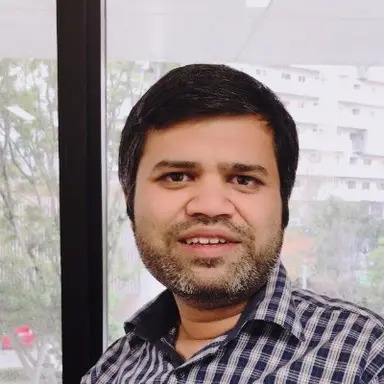
LLM-Based Reasoning: Opportunities and Pitfalls
The question of whether Large Language Models (LLMs) can reason is both complex and crucial. While LLMs have demonstrated capabilities in informal reasoning, their potential extends far beyond, particularly in advancing explainable AI and the development of intelligent agents. In this talk, I will explore the reasoning abilities of LLMs and discuss the ongoing efforts at IBM to enhance these capabilities in IBM granite models by using knowledge and tool-assisted synthetic data generation.
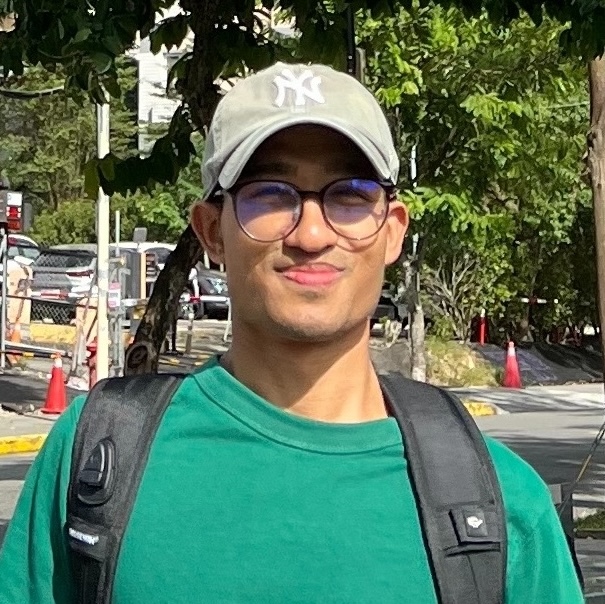
Adversarial Attacks and Defenses on Vision-Language Models
Robustness research aims to build machine learning models that are resilient to unpredictable accidents (black swans and tail risk robustness) and carefully crafted and deceptive threats (adversarial robustness). In this talk, I shall focus on the adversarial robustness of vision-language models (VLMs). Specifically, I shall cover recent attacks and defenses on two kinds of VLMs: vision-language pre-trained models like CLIP and vision-conditioned LLMs like LLaVA. The talk will focus on discussing whether existing attacks are practical and what the challenges and opportunities of adversarial machine learning research are in the space of vision-language models.
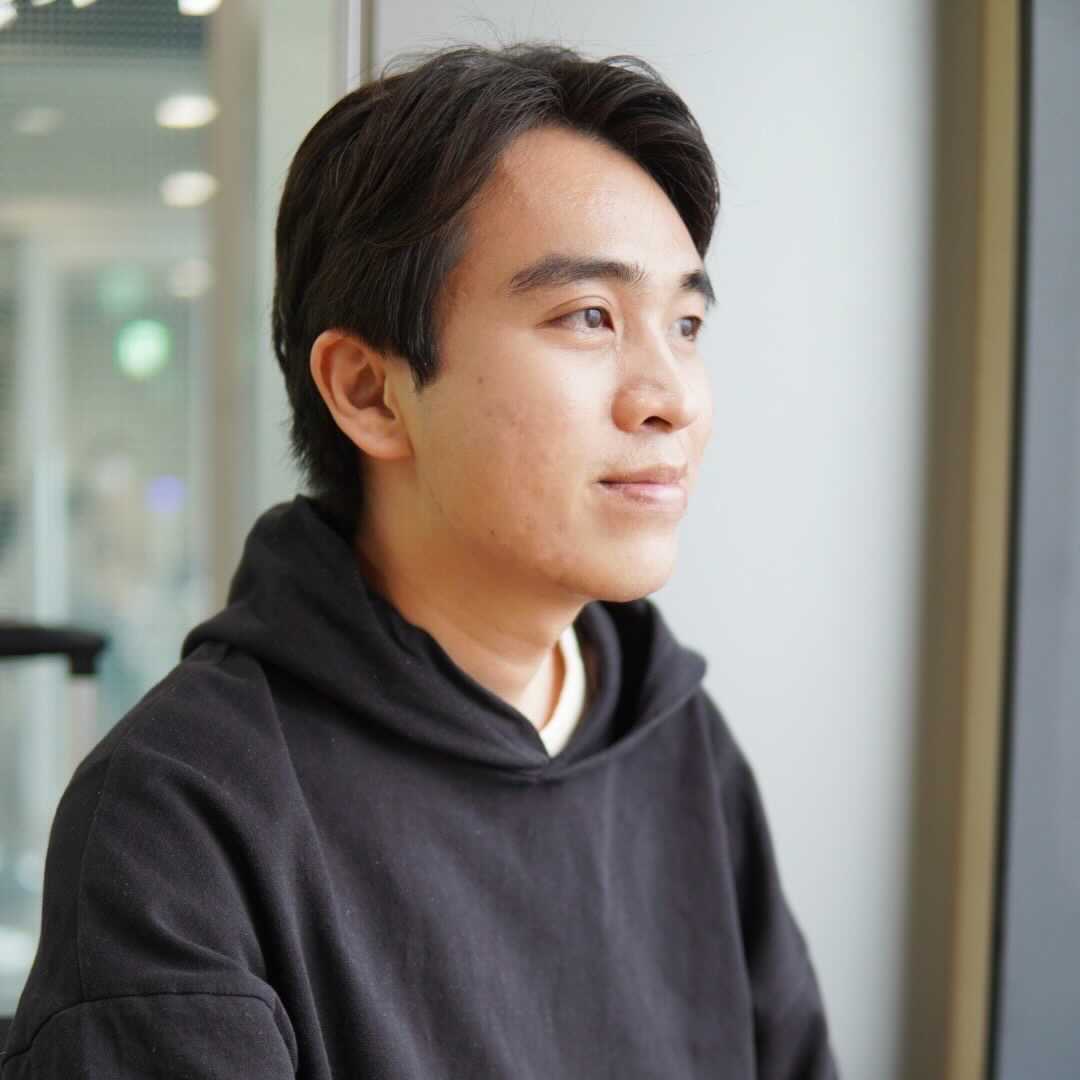
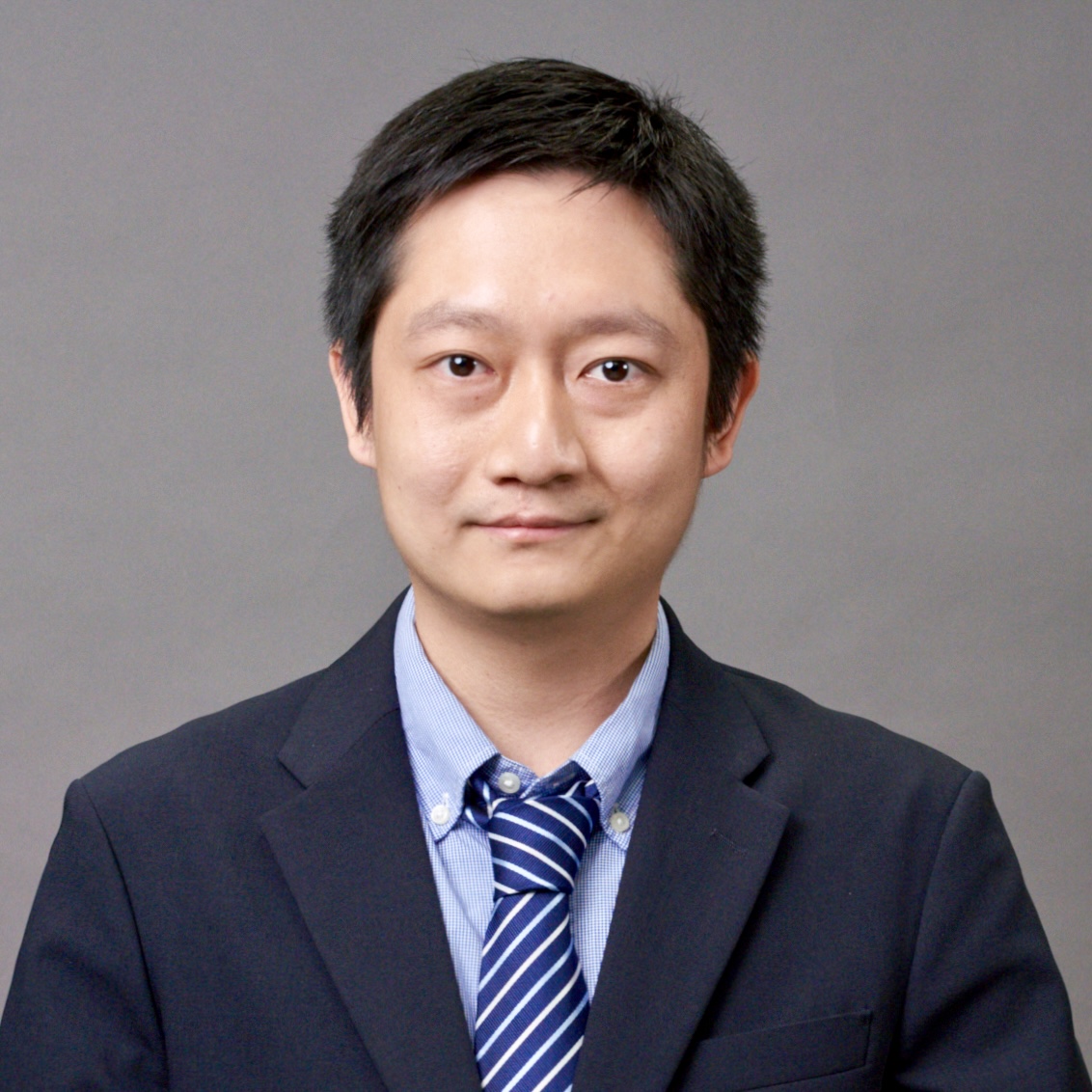
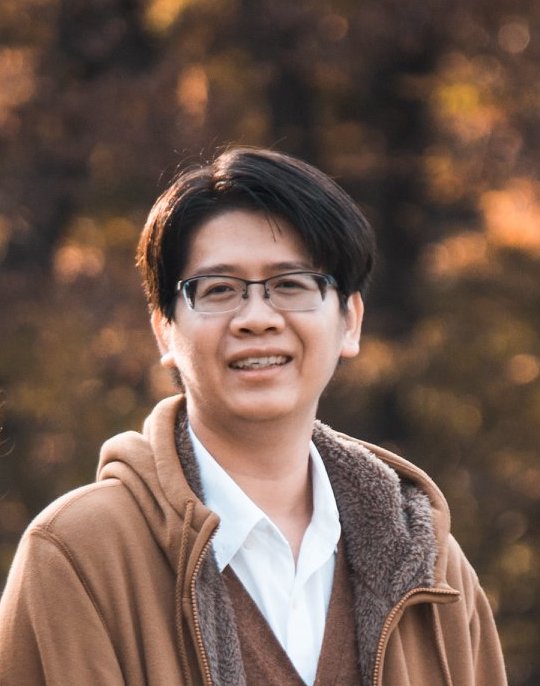
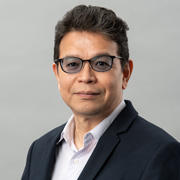
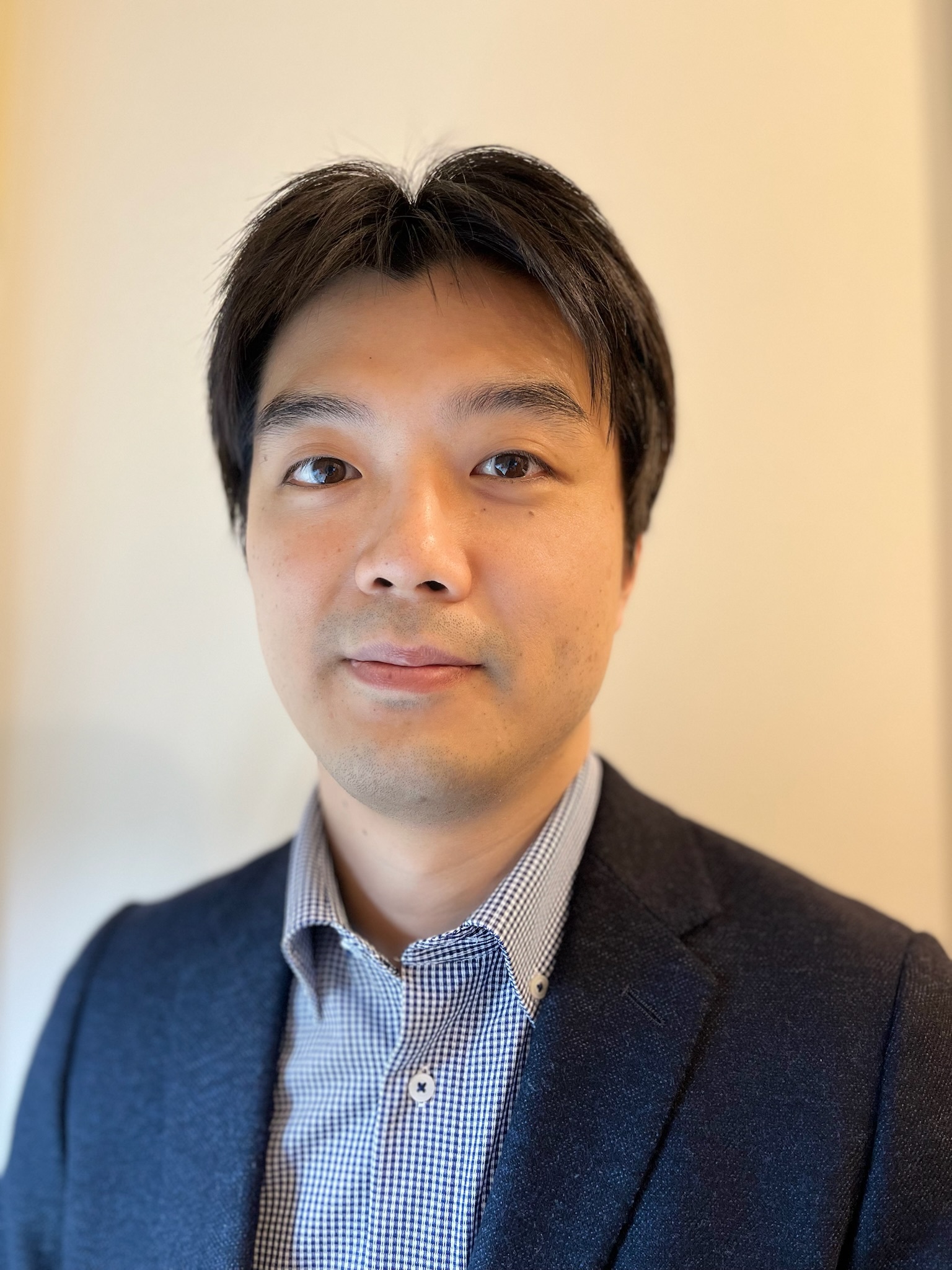
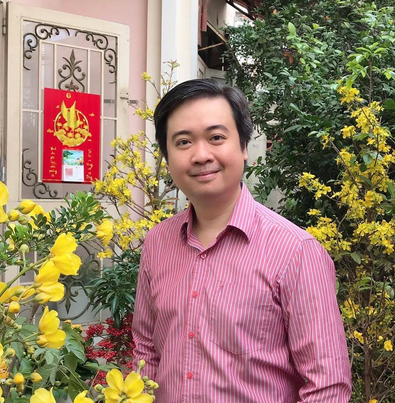
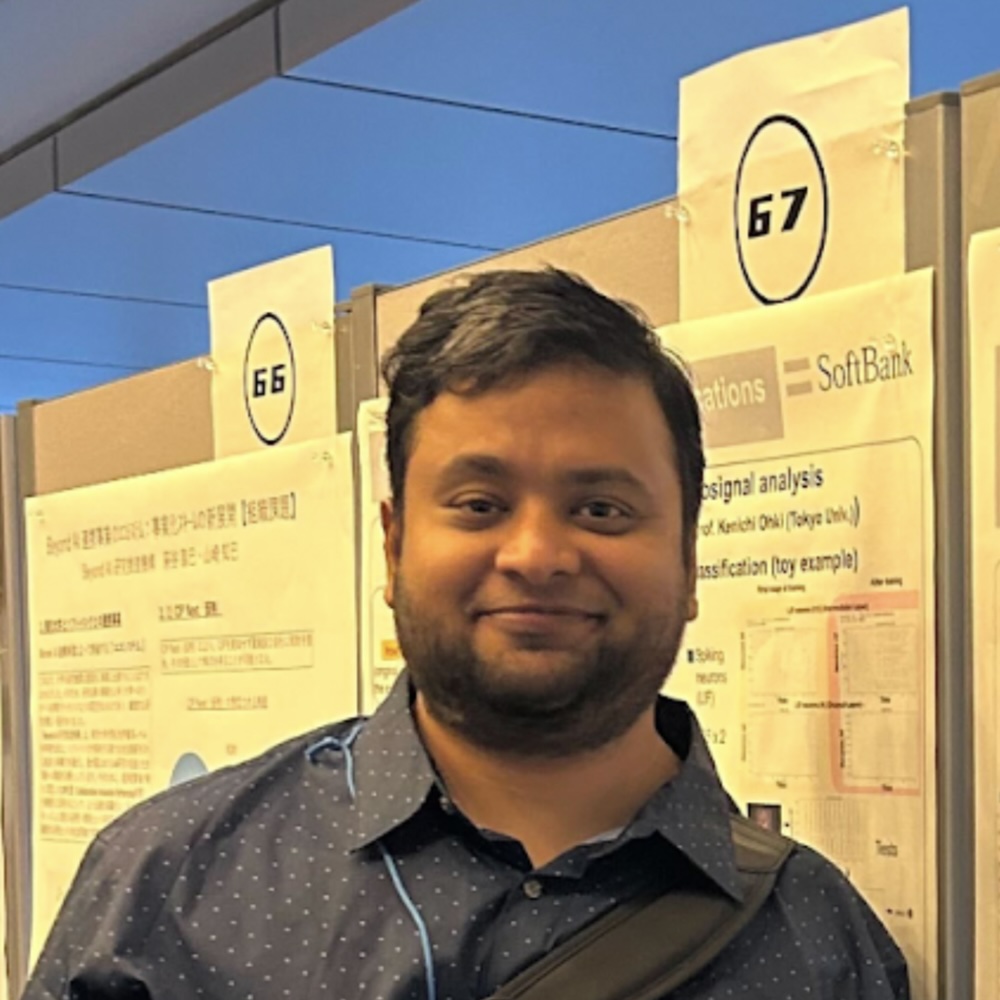
Contact: lava-workshop(at)googlegroups.com
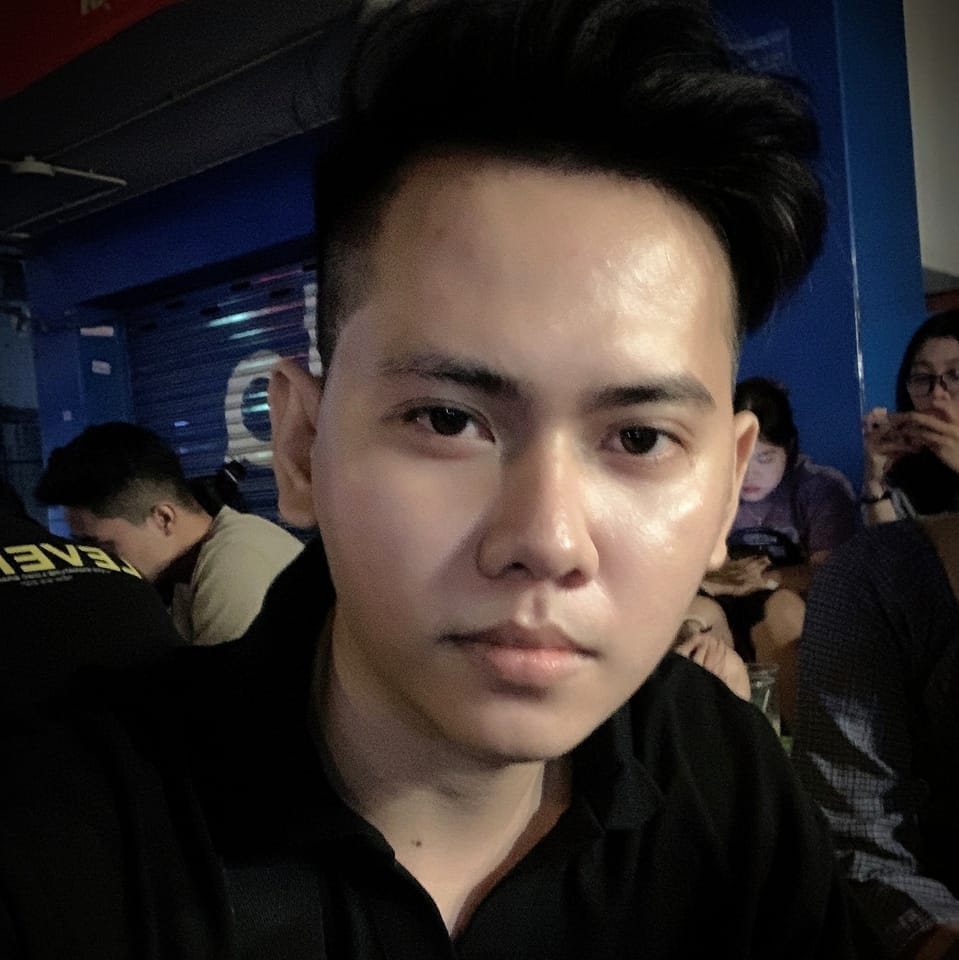
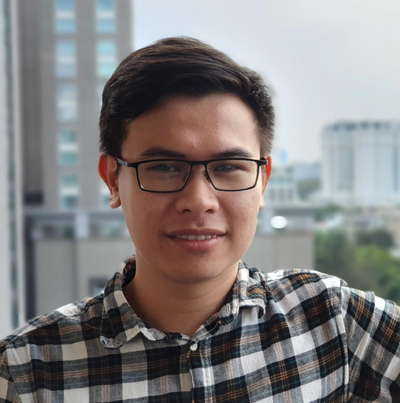
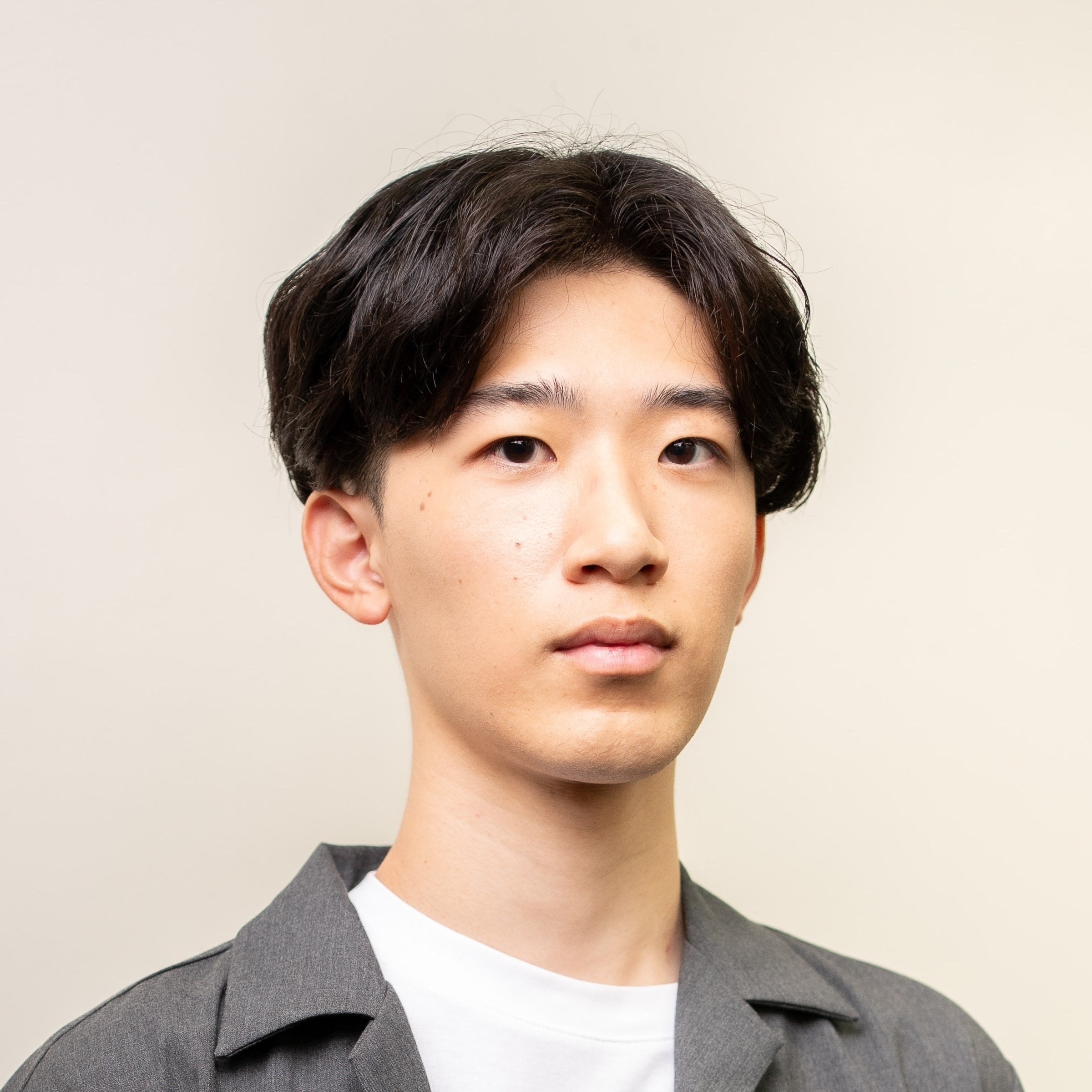
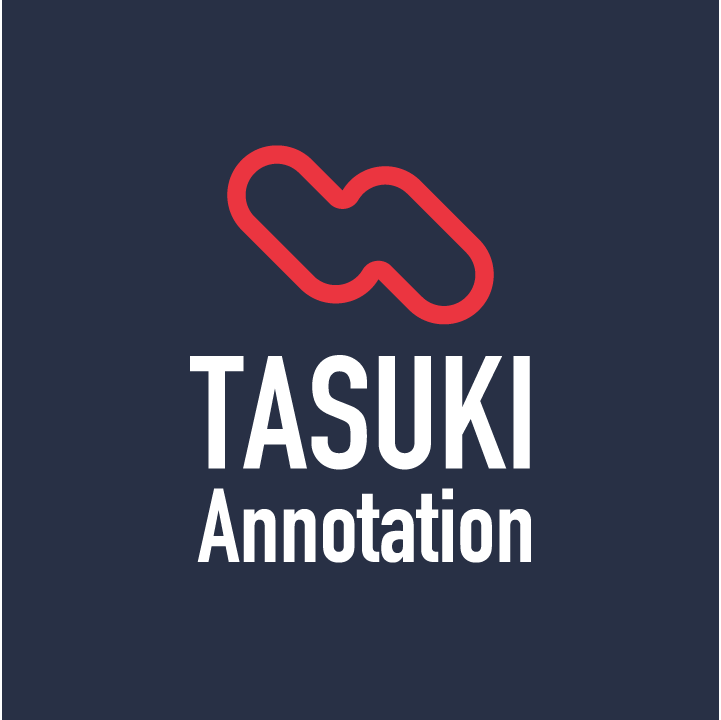
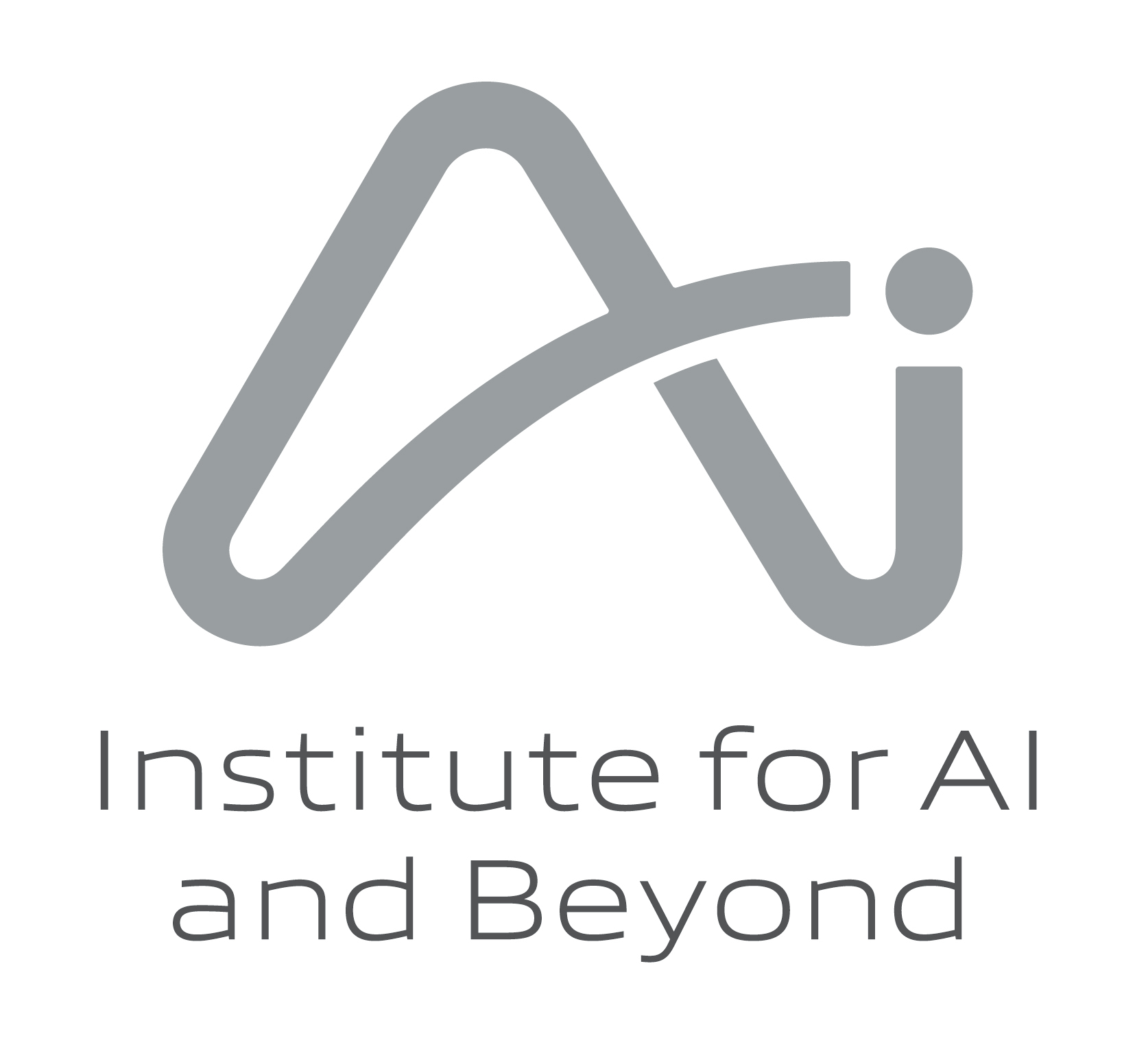